How do regular meteorologists forecast the weather? In this two-part series, we will compare conventional practices to The Old Farmer’s Almanac methodology. It’s not the data that we collect; it’s how we use it! Let’s start with Part I: Conventional Weather Forecasting.
Part I: How Conventional Forecasts are Made
Predicting the Weather—From Days to Hours Ahead
Most of the weather forecasts that we see on TV or the Internet or hear about on radio cover the period from the next few hours to the next few weeks.
The shortest-range forecasts, also known as nowcasts, typically cover the next hour or two, and are focused primarily on precipitation—especially the exact starting and ending times and intensity of any rain, snow, and ice that will occur.
As you might expect, the most important tool used for these forecasts is radar, which shows the current precipitation and how it has been moving and changing in intensity. To a large extent, these forecasts take what is happening now and how it has changed over the past hour or so and extrapolate these events into the next couple of hours.
Lightning strike data and high-resolution satellite imagery provide additional insights into this extrapolation, while computer-generated forecast models suggest changes beyond pure extrapolation of trends.
Forecasts covering the next 1 to 10 days or so are based largely upon computer-generated, deterministic forecast models, which start with the current state of the atmosphere and use the physics of fluid dynamics (after all, the atmosphere is considered to be a fluid) to predict how the atmosphere and its weather will change.
Beyond about 10 days, the computer models rapidly lose their ability to make precise forecasts because their errors tend to grow and compound over time. Small errors in the initial state of the atmosphere (even those as small as half a degree in temperature or one mile per hour in wind speed) can sometimes grow over time within a computer forecast model, assuming an unwarranted significance that drives the forecast to a substantial error.
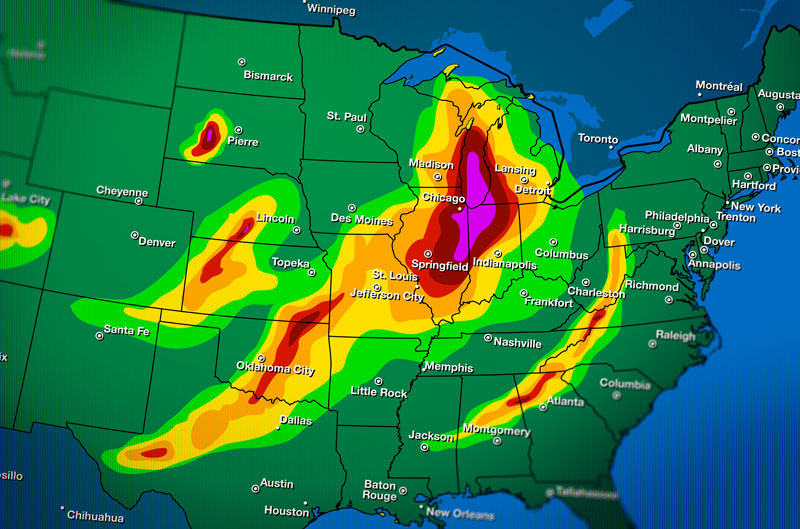
Image by SpiffyJ/Getty Images
The Problem of the Microscale
As weather professionals, we do indeed have detailed weather information from physical observations, sensors, radar, and satellite imagery. However, we do not have this information on a microscale; our initial data does not include the variations caused by a stream running through a farm or wind that is funneled between large buildings. Plus, we do not fully understand all of the driving forces within the atmosphere, such as the microscale physics that form individual clouds and tornadoes.
For these reasons, conventional forecasters parameterize the microscale effects, meaning that they use simplified equations that capture these developments well enough in the short term but may introduce errors that compound and grow over time.
Even though computers continue to get faster and more powerful, their capabilities are not infinite, and weather forecasting is one of their most complex and intensive uses. If it took a computer 48 hours to produce a forecast starting 2 hours hence, that forecast would be of no value, so some additional simplifications need to be made in the forecast models to enable them to generate timely forecasts.
The small errors in initial conditions and simplifications in the physics that are necessary because we have neither infinitely accurate initial data nor infinitely powerful computers were the inspiration for the concept of a so-called “butterfly effect”—that, for example, something as simple as a butterfly flapping its wings in central America can create a tiny change in the local wind flow that can grow and amplify over time to result in a hurricane hitting the Gulf Coast a week later.
One way in which scientists account for this is by running the computer models multiple times with small changes in the initial weather conditions—for example, we would change the temperature from 78.2° to 78.4° and see how the forecast differs. Known as ensemble model forecasts, these run-throughs can show weather professionals the full range of possibilities and give an idea of their probabilities that deterministic models cannot.
Once we get beyond about 20 days, the errors in computer-generated forecasts compound to the point where they are no more accurate than climatology and lose their usefulness. So, for longer-range forecasts, conventional forecasters need to utilize different strategies.
See “Part II” to compare “How the Almanac’s Forecasting Methodology is Different.”